%20(1080%20x%20551%20px)%20(1080%20x%201080%20px)%20(10)%20(3).png)
Revolutionizing Waste Management with Advanced Data Solutions
Enhancing Recycling Efficiency Through Precise Data Annotation
Services
Data Engineering
BI & Data Analytics
AI & Automation
Data & AI
Company Background
Glacier Robotics was founded with a mission to revolutionize waste management's impact on climate change and environmental conservation. Recognizing that half of the recyclables in the U.S. end up in landfills, the company aspires to increase recycling rates, reduce pollution, and conserve natural resources. By supplying more recycled feedstock for manufacturing, Glacier Robotics contributes to energy-efficient production processes, thus cutting down greenhouse gas emissions. With a visionary goal of driving a circular economy and achieving 10 billion tons in global greenhouse gas reductions, the company stands at the forefront of transformative solutions in waste management. They reached out to us to tackle a critical challenge in their quest for an efficient and accurate recycling platform. Faced with the complex task of labeling and categorizing over 80,000 images of recyclable products within a tight timeframe, they sought our expertise in data annotation and verification.
.png)
Embarking on a time-sensitive initiative, Glacier Robotics presented us with a formidable task — labeling an extensive set of 80,000 recyclable product images in a mere two weeks. The urgency stemmed from the company's mission to train their robots for precise waste sorting in recycling plants. Adding to the complexity, the labeled data required meticulous verification, either through sophisticated data engineering or randomized testing, ensuring the integrity and quality necessary for subsequent model training.
Goals of the Project:
This ambitious undertaking comprised three key objectives:
Efficient Data Labeling:
Swift and accurate categorization of 80,000 recyclable product images, a critical step in training robots for effective waste sorting.
Verification System:
Development of a specialized machine learning model to systematically verify the accuracy of the labeled data, ensuring reliability in subsequent stages.
Timely Delivery:
Adherence to a stringent two-week timeframe, aligning with the project's time constraints and emphasizing the need for expeditious completion.
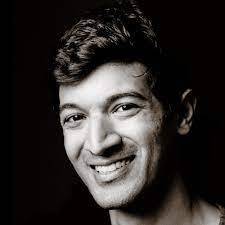
Project Onboarding: Our onboarding strategy prioritized privacy, abstaining from collecting personally-identifiable information. Demographic inputs, crucial for meaningful data analysis, were solicited within ranges carefully structured to provide precision while preventing unwarranted profiling.
User Anonymization Preferences: Users, during onboarding, selected their preferred level of anonymization via a slider, influencing the granularity of recorded location, demographic, and symptomatic data. This innovative approach gauged user comfort and willingness to share information.
Daily Health Questionnaire: Initiating with an initial health questionnaire, users received daily prompts via browser or push notifications, ensuring routine engagement. The questionnaire's efficient design and multiple-choice format streamlined data collection, expediting both user interaction and subsequent analysis.
Map-Based User Dashboard: Our user-centric dashboard offered two map-centric views centered on the user's location. One displayed COVID-19 case data from Johns Hopkins University, providing a national to county-level perspective. The other, a 'viewfinder' experience, depicted users reporting symptoms within the visible area, maintaining privacy through differential privacy measures.
Differential Privacy Integration: Implementing differential privacy safeguarded user location data, providing anonymity to data points while ensuring its utility for analysis. Users had the flexibility to choose their privacy level, striking a balance between personal privacy and data usefulness.
Technological Landscape: Selecting Flutter for mobile and React for the web, we prioritized cross-platform efficiency and ease of development. The web server, built on NodeJS, delivered a seamless user experience. MongoDB, chosen for its flexibility, served as our database, while Python, with NumPy and Pandas, powered the data server, managing processing, updates, anonymization, and backups.
Infrastructure and Content Management: Leveraging AWS EC2 infrastructure, our servers and web applications were meticulously developed. Additionally, a WordPress application facilitated easy content editing for static pages, enhancing the platform's agility in delivering information.
Our approach aimed not only for technical excellence but for a user-centric, privacy-respecting solution that aligned seamlessly with Glacier Robotics' mission in revolutionizing recycling practices.
Efficiently labeling 80,000 data points within the stipulated 10-day period, we successfully created a reliable machine learning model for data verification. The well-structured output and database provided a foundation for further training the trash-sorting robot, refining its accuracy.
In a transformative partnership with Glacier Robotics, we achieved remarkable milestones in the realm of waste management and recycling technology. Through strategic alliances and the implementation of a cutting-edge internal verification model, we not only met but exceeded expectations, culminating in the successful deployment of innovative trash-sorting robots across multiple locations in Northern California.
Labeled 80,000 recyclable product images in just 10 days.
Implemented a robust ML model for meticulous data verification.
Enabled the deployment of trash-sorting robots in 10 pilot locations and two large-scale commercial setups across Northern California.
Achieved a seamless integration of labeled data, contributing to the enhanced accuracy and efficiency of the deployed robotic systems.